From 0 & 1 to A - Z: What data is really worth
How to successfully implement AI
in your business - Part 3
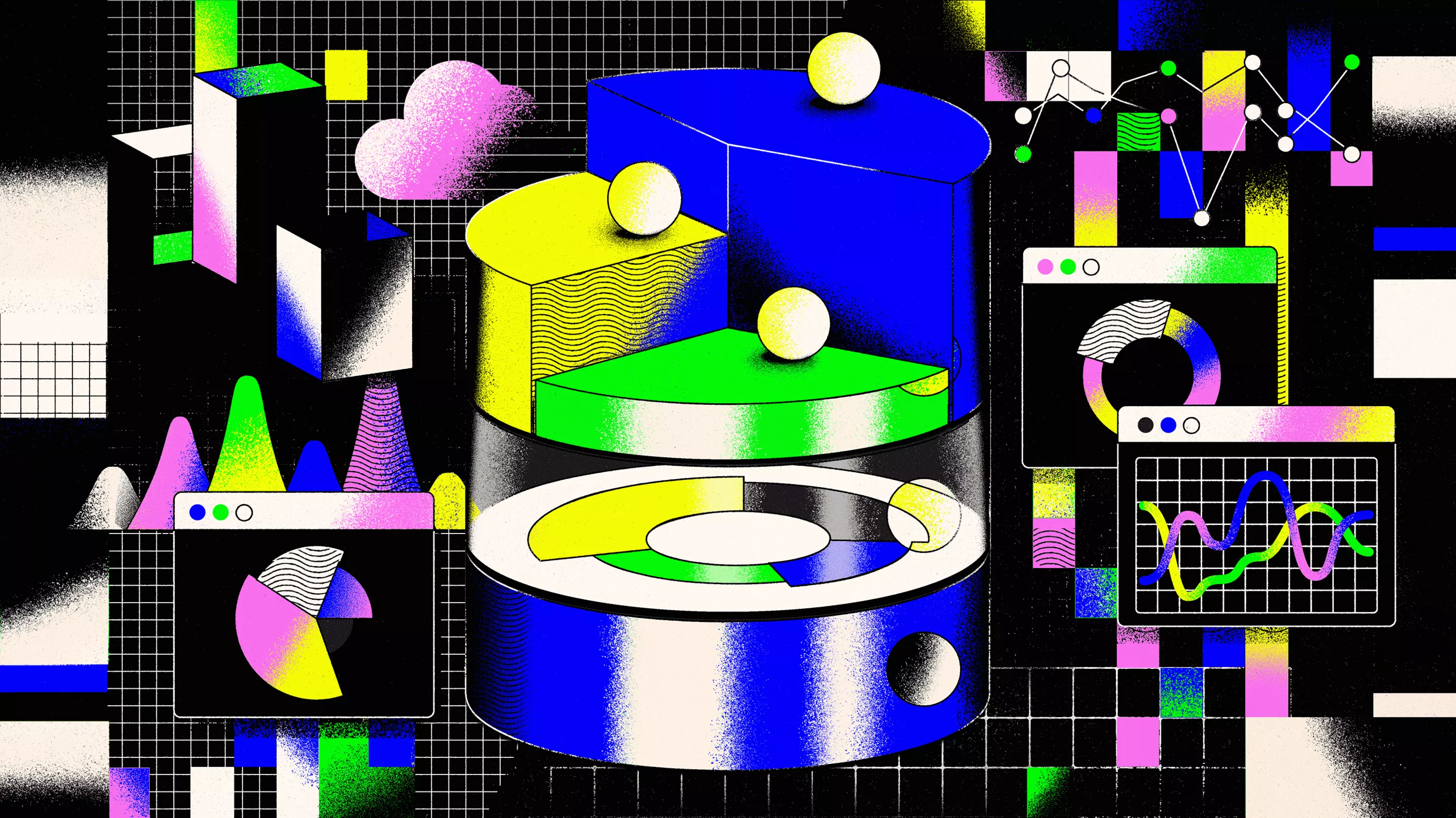
Yes, on principle you have decided to use artificial intelligence at your company. And also, yes, you have already envisaged a concrete use case that is precisely aligned with your corporate strategy. With that, you have already taken the first two big steps. The next step is to get a realistic assessment of the feasibility of your desired AI. What resources do I need for successful implementation? What know-how do I need to implement it? What (follow-up) costs will I incur?
In my last newsletter, I showed that using AI in companies can only be successful - that is, beneficial and profitable - if the necessary data availability, technical feasibility and economic viability are taken into account.
In this article, I would like to take a closer look at one of these factors: data availability. What is data, actually? What distinguishes "good" from "bad" data? How can you obtain it? And what makes it so valuable - for your AI project and for your company?
If you want AI, you need data
I often have to think of Niki Lauda. When asked for his recipe for success, the three-time Formula 1 champion once answered that he was the first to recognise that it is not the best driver who wins the race, it is the fastest car.
A captivatingly clear sentence that, for me, names the fundamental factors of every successful enterprise: momentum, insight through a change of perspective and the expansion of relevant resources. You remember:
In the first article in this series, we argued for the relevance of AI for your business today (momentum). In the second article, we identified various approaches for how to identify the most suitable applications of AI for your business (a shift in perspective). Here - you guessed it - we want to talk about the defining resources for your AI model: your data. More precisely: how you can check, secure and expand the availability of the relevant data.
What is data, actually?
Let’s stick to the metaphor: Data is the fuel for your AI engine. The basis of all digital processes. The raw material from which AI can bundle information, make calculations and optimise processes in the first place. Data is not just zeros and ones, it is the A to Z for your AI - and for your business model.
The good news first: every company, including yours, has masses of data. The older the business, the more data is potentially available. From files, contact addresses, support emails, support phone calls, instructions, product information to internal wikis, images, videos, financial, stock market and weather reports - these are all data sources that can be analysed and made useful for the company. But how?
How data becomes valuable
Taken on its own, in isolation, data has no meaning and therefore no value. Just imagine a collection of numbers and letters on a sheet of paper. Mere characters. Only when they are organised and readable according to a general pattern, a syntax, you come to realise that what you’re looking at is, for example, a telephone list including telephone numbers, call time and date. Characters become data.
If you now place this data in the context of other data of your business activity - for example, frequently asked questions at your service hotline - you will gain new insights into these fields of activity. For example, who asks which questions to your hotline and when. Information is created by linking data and context.
If you integrate this information into your company's existing network, such as the human resources department, and in turn connect it with other information, say from product instructions, knowledge bases, etc., new insights can be derived, for example, for personnel deployment planning and qualification of hotline staff. Information becomes knowledge.
If your company acts on the basis of this "knowledge" for some time, it gains "experience". On the basis of this experience - i.e. the collection and consolidation of data over a longer period of time - predictions can be derived for the future regarding recruiting, deployment planning and procurement. The data is used in the most efficient possible way.
A practical example
The following case study shows how accurately AI can make predictions by combining data and can thus optimise processes. We developed an AI-based cost forecasting tool for an insurance company that provides employees with a quick and reliable basis for making decisions in the event of a claim.
So, we first analysed the insurance company's collected data, its "wealth of experience", namely the claims reports of the past ten years. On this basis, we combined our client's expert knowledge of motor vehicles with the insurance company's individual control rules. The AI model we developed was able to provide service staff with faster and more precise cost forecasts based on this data linkage.
The result: optimised claims management, reduction of claims costs, and more satisfied customers and employees.
Good data, bad data
It sounds simple, but it isn’t. Yes, AI can link and combine data in a fraction of a second. But in order for the linkage to produce accurate predictions, you need more than just the right amount of data. The data quality must also be a given, in order to avoid erroneous conclusions within the many derivation processes. The results of an AI are only as good as the data from which it has learned.
In essence, the more structured and error-free the data is, the easier and more accurate the AI’s automatic processing and evaluation will be. What counts in the end is the actual benefit of the application. Thus, the following minimum requirement must be applied as a rule of thumb: the desired data quality is only achieved when a targeted use case can be implemented with the existing data, without errors.
What you need to pay attention to
Before an AI can function or deliver reliable results, a solid, clean data foundation must be built, or must at least be available in the foreseeable future. How do you manage that?
Here is a Top 5 hit list of data aspects to consider:
1. Incomplete data:
Inaccurate numbers, missing data fields, limited time periods or data resolutions can affect the quality of the training of the AI. You must check whether all the necessary data points to implement your AI model are available or at least potentially available.
2. Incorrect, biased or ambiguous data:
Non-representative or incorrect data sets distort the results of AI models. Skewed, unbalanced data ratios can lead to ethically unacceptable and/or factually unfounded conclusions that result in, for example, discrimination against certain groups of people.
3. Data quantity:
Too little data can make AI training ineffective, while too much data becomes unwieldy, leading to scaling problems and requiring disproportionate computing capacity. For guidance, depending on the application, around 10,000 to 500,000 data records is a sufficient order of magnitude. If the necessary balance is not given, improvements have to be made, for example through new readouts or synthetic generation.
4. Data protection and data security:
In order to ensure compliance with regulations, special care is required when handling confidential, personal data and otherwise sensitive data. Wherever, for example, bank data, personal data of employees, asset information or information on medical conditions are used, regulatory and IT security know-how is required.
5. Non-standardised data:
Data from different sources or in proprietary formats require more complex procedures for reading and standardisation. For example, Microsoft Word documents, continuous text from PDF files, file formats of special industry software or AutoCAD drawing. This can sometimes mean more than just overtime for your data engineers and developers.
Data as enterprise value
Please, understand me correctly: these are not reasons to bury your head in the sand and not deal with data, quite on the contrary. In order for the introduction of AI in your company to be successful, I believe it is essential to consider these points in advance. They are all part of a realistic resource, cost and benefit calculation. Only when you have an overview of the field of data availability can you unleash the full potential of your AI model and your company.
Collecting, mining or procuring data
After all, the world of data that is relevant for you doesn’t only exist within your company. There are ways to acquire missing data sets, or gather new ones. You can buy external data sets (the EU Data Act is opening completely new horizons) or you can generate them synthetically with the help of AI. Through data analytics, you can mine existing data sets, or use new products to collect new data. You already have some of the data that you need, while other pieces will be available to you in future. But certain data will simply not be available or cannot be obtained. You also need to be clear about this aspect. The point is: at the latest when you are dealing with AI, you should know that data is your most important resource. And deal accordingly with the availability, nature and procurability of your data. Think of Niki Lauda.
Some say that data is the new gold or the new oil - I don’t believe in these kinds of comparisons. Gold and oil are limited natural resources and (as far as I’m aware) cannot be reproduced. If you use oil, you burn it and use it up. If you own gold, you don’t use it, it stays stored away. The value of both resources lies in their scarcity. The less there is, the higher the nominal value, and the better for its owners.
With data, it is fundamentally different. The more data a company owns, the more valuable it is. And it becomes more and more.
That is why data is also more valuable to companies in the long run than the AI algorithms that interpret it. An AI model can become outdated, or overtaken by new approaches and technologies. But the data, once it has been collected, remains, and becomes an ever more powerful raw material for ever more complex models.
The ace up the sleeve of SMEs
And therein also lies the tremendous potential for SMEs. With decades of experience in the market, medium-sized companies have a decisive competitive advantage over the start-ups that challenge them: their wealth of data. Every startup, by definition, lacks this "experience" - as translated into data sets. But this is what they need, against all odds, to train their models and validate their cases. This expertise of "experienced" industry oldies cannot be replicated by any AI that has only been trained on the basis of a limited extract of the internet. Companies thus have a tremendously valuable resource with which they can train their own AI models and secure decisive competitive advantages.
I am convinced that SMEs currently have the best cards in their hands. Now it's a matter of playing them right. The momentum is on your side. Within an industry, often only the first movers can really exploit their data and use their advantage strategically. For example, you could sell the AI models developed from your own data as licensed software to other companies in other industries. Momentum, Niki Lauda, you know what I mean ...
Human Resources
You see: Whatever door you want to open with your company - data is the key. But even though we are surrounded by it everywhere, data does not fall from the sky. Data must be stored, analysed, prepared, maintained and processed. For this, you need know-how and the corresponding human resources.
Niki Lauda had it comparatively easy in this respect: he relied increasingly on designers, engineers and mechanics - in other words, everyone who could build him a fast car. When setting up your data team, it is important to have the right specialists and disciplines on board, depending on the AI requirements and project phase - data scientists, data analysts, data engineers, developers, consultants or project managers. Whatever your strategy, make sure you put your valuable assets in good hands.
What's in your data?
Finally, the good news again: Yes, you too have masses of data! But do you also know its value? If you had gold in your cellar, I bet you would have analysed, counted, catalogued and saved it long ago. But data treasures still lie unmeasured and unused in the dusty databases of many companies. This cannot and will not last for long.
At the latest when the first Niki Lauda of their industry appears, these companies will be relegated to the back ranks. Don't wait until that time comes. Use your enormous data potential and put your company in pole position. Otherwise, others will pull ahead.
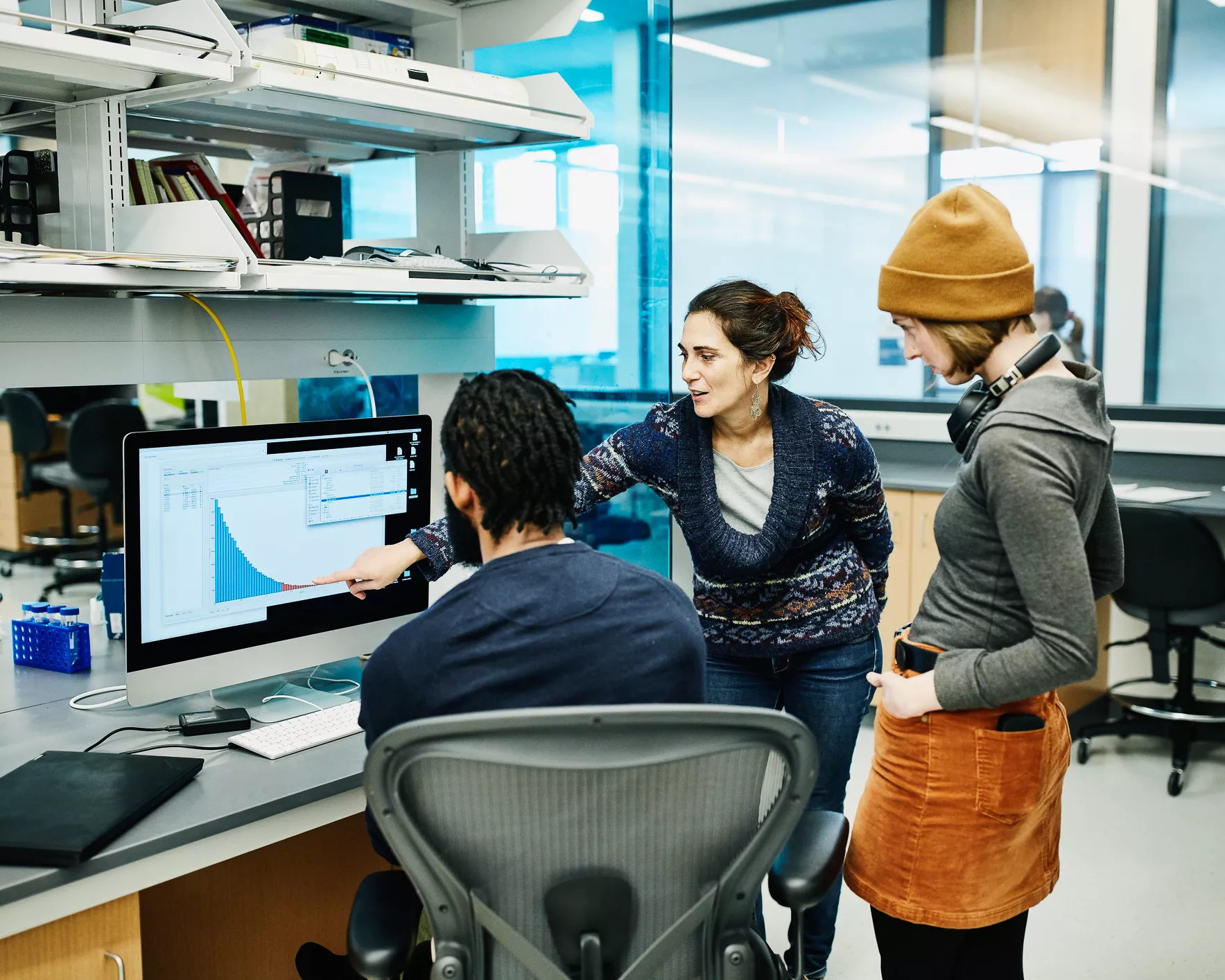
So that you know what treasure trove of data you are sitting on, we at PLAN D offer a practical solution: Within ten days, our data scientists will carry out a targeted analysis and evaluation of your data sets. Whether it's process optimisation, compliance with data protection guidelines (such as anonymisation of sensitive data) or the maximum exploitation of existing data values - we prepare your data so that you can work with it. Quickly, securely and thoroughly.